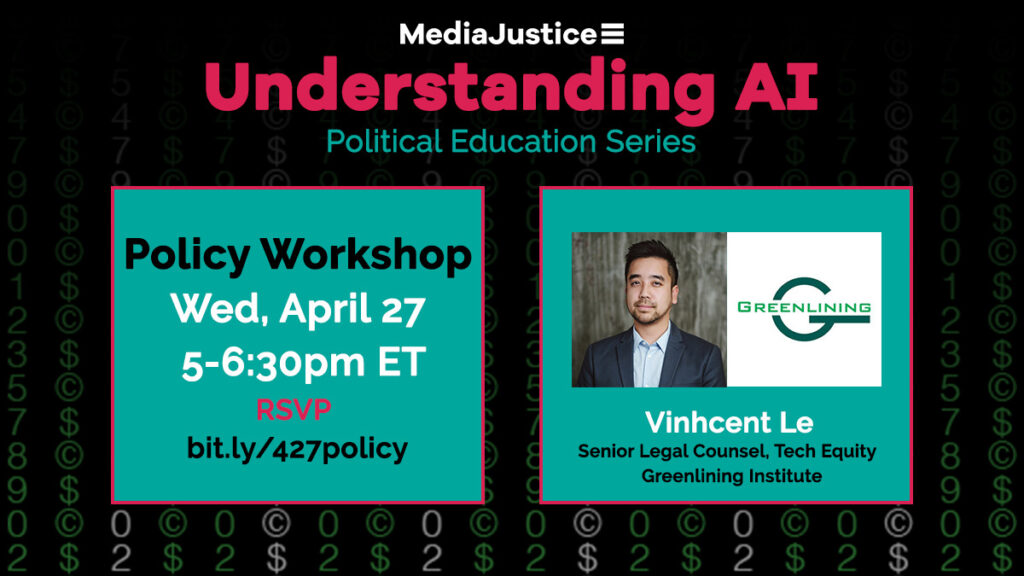
AI is more than C3PO and Alexa. Artificial Intelligence and algorithms are becoming universal. However, AI can create unfair, biased outcomes that can limit what job you get, the school you go to, whether you can buy a home, and more. As part of our Political Education Series, we have the pleasure of learning from those fighting for unbiased, equitable outcomes for marginalized communities in the digital age.
Leading the second part of our series is Vinhcent Le, Senior Legal Council from Tech Equity Greenlining Institute. We had the opportunity to talk with Le about his thoughts on his report “Algorithmic Bias” and his efforts in working towards equitable financial opportunities today.
Dulani: Tell us a little bit about the Greenlining Institute and what motivated you to write a report on Algorithmic Bias.
Vinhcent: The Greenlining Institute is full of advocates like me working towards a future where communities of color have full access to economic opportunity and are ready to deal with the challenges of climate change. Founded in 1993, we are focused on undoing ‘redlining’, a discriminatory practice that puts services (financial or otherwise) out of reach based on your race or ethnicity, and replacing it with ‘Greenlining’, the proactive work of bringing investments and opportunity to communities of color.
While our work covers many policy areas – my focus is on the intersection of economic opportunity and technologies, such as algorithms, A.I., and the Internet. With regards to algorithms and A.I., I investigate redlining and how these tools can recreate discrimination on a wide scale. I was motivated to write the Algorithmic Bias report because when I spoke to folks outside of the technology industry, I regularly heard the following questions:
- What are algorithms and where/how are they used?
- Algorithms are data-driven, how can they be unfair or biased?
- How does it affect me or my constituents/what are the impacts on communities of color?
- What are our existing laws and rules around algorithms?
- What do we do about algorithmic bias?
I wrote “Algorithmic Bias Explained” intending to create a resource to answer those questions. You’ll see that it (hopefully) provides an accessible introduction to algorithms that answer the above questions. Please give it a read!
For folks not familiar with Algorithmic Bias, how would you both define and demystify what it is?
Algorithmic bias arises when algorithmic decisions unfairly or arbitrarily disadvantage one particular group or another. The algorithmic bias I’m most concerned about comes from “automated decision systems” —replacing human decision makers like judges, doctors, bureaucrats, or bankers. These algorithms take our data (i.e. age, gender, income, education, zip-code etc.), and process that data according to a set of rules, generating an output that becomes the basis for a decision. For example, a banking algorithm could process your loan application by looking at thousands of creditors with similar characteristics to you and generate an output predicting whether or not you would default on a loan – the bank would then use that prediction to approve or deny the loan.
The set of rules an algorithm uses to make a decision often comes from “training data”. This data can consist of thousands of past examples of how humans made decisions. Essentially, an algorithm can “learn” how to make decisions by finding patterns in the training data. For a bank, this could mean a dataset consisting of all the loan decisions that human bankers made in the past. The problem with this type of machine learning is that the training data may reflect human bias which the algorithm will then learn and replicate. In our above example, a banking algorithm that learns from historical credit decisions will likely learn to discriminate against people of color as the practice of racial redlining is baked into that data.
Where and how does Algorithmic Bias show up in everyday life? How are marginalized communities uniquely impacted by it?
Algorithmic bias can show up often in everyday life in invisible ways that result in a minor inconvenience or a major obstacle. For example, price optimization algorithms can use your internet history to decide whether you get a coupon on a pair of shoes you left in your cart. On the other side of the spectrum, a similar price optimization algorithm used by a university may reduce the financial aid or scholarships you get by tens of thousands of dollars, because the data shows students in your zip code are likely to enroll even with minimal financial aid.
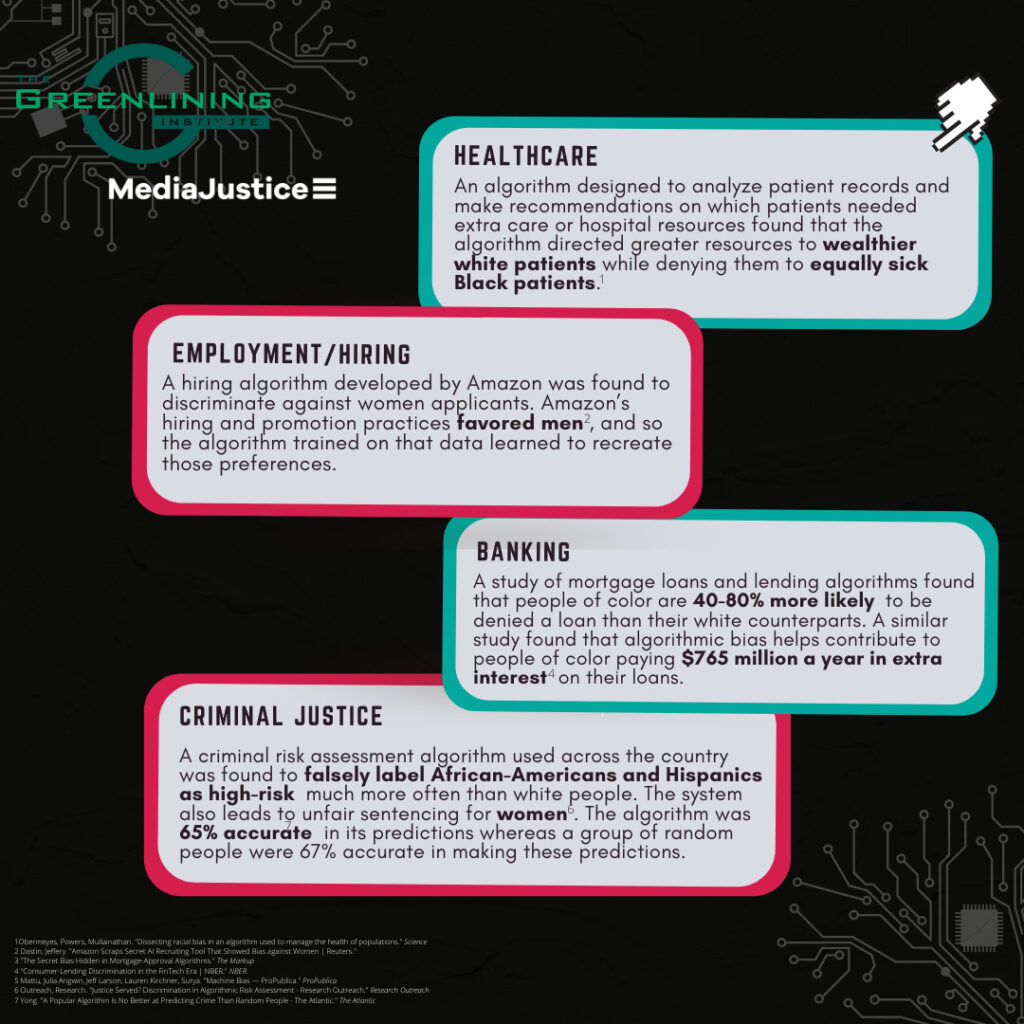
- Employment/Hiring:
- A hiring algorithm developed by Amazon was found to discriminate against women applicants. This was attributed to the fact that Amazon’s hiring and promotion practices favored men, and so the algorithm trained on that data learned to recreate those preferences.
- A hiring algorithm developed by Amazon was found to discriminate against women applicants. This was attributed to the fact that Amazon’s hiring and promotion practices favored men, and so the algorithm trained on that data learned to recreate those preferences.
- Medical Care:
- One major healthcare algorithm was designed to analyze patient records and make recommendations on which patients needed extra care or hospital resources. Researchers found that the algorithm directed greater resources to wealthier white patients while denying them to equally sick Black patients.
- One major healthcare algorithm was designed to analyze patient records and make recommendations on which patients needed extra care or hospital resources. Researchers found that the algorithm directed greater resources to wealthier white patients while denying them to equally sick Black patients.
- Banking:
- Criminal Justice:
- A criminal risk assessment algorithm used across the country was found to falsely label African-Americans and Hispanics as high-risk much more often than white people. The system also leads to unfair sentencing for women. The algorithm was 65% accurate in its predictions whereas a group of random people were 67% accurate in making these predictions.
As you can see, algorithmic bias is present across many different industries and sectors of our society, but is often overlooked because of the lack of resources to identify when an unfair algorithm has impacted them. Marginalized communities typically feel these impacts the hardest. The historical discrimination their parents and grandparents faced is baked into the data and is used to make decisions about them today. Beyond that, the lack of diversity in the tech sector amplifies the risk of implicit biases by the coders and developers. Those biases get encoded into key decision making algorithms, while the perspectives and experiences of marginalized communities are ignored.
What opportunities and actions can people take to combat Algorithmic Bias?
Spread the word! This year, the U.S. Senate introduced the Algorithmic Accountability Act which would require companies to check if their algorithms work as intended or contain bias. Beyond that, over twenty states have introduced data privacy legislation and nearly half of those include protections against automated decision making and AI. This is progress!
When I first began working on this issue over five years ago, the idea of algorithmic bias was not widespread. I, like many others, thought that more data and technology would mean a fairer, more equitable society. Technology companies had free reign to develop and deploy these algorithms with minimal oversight and major impacts on society. Today, that is beginning to change. Our elected officials and regulators are just beginning to realize that these tools are not perfect and algorithmic bias is real. However, many have been convinced by the technology industry and their lobbyists that algorithmic discrimination is a minor problem that can be fixed internally and that protections against algorithmic bias would cripple “innovation.” This perspective ignores the real human costs that occur when these systems are biased and inaccurate. It gives us the idea that we have to tolerate inaccurate algorithms in the name of innovation. That’s not true! I truly believe that we can have the best of both worlds and the companies and governments deploying these algorithmic tools have to be responsible and actually test if these systems are working fairly and as intended – something they’re not required to do today.
So the biggest thing you can do right now is to contact your representatives locally, at the state level, and at the federal level to make some noise about this issue. Let them know this is something you care about. This contact can help build the pressure necessary to pass AI legislation, requiring the government and large businesses to test their algorithms for bias or report on what steps they’ve taken to minimize the harms I’ve discussed above. Without grassroots support from the public, it will be tough to get the accountability and oversight we need to minimize and eliminate algorithmic bias.